The Young Investigator Network is the platform and democratic representation of interests for independent junior research group leaders and junior professors at the Karlsruhe Institut of Technology.
Peer Community
Representation of Interests
Professional Development
Academic Leadership
Individual Support
Funding Opportunities
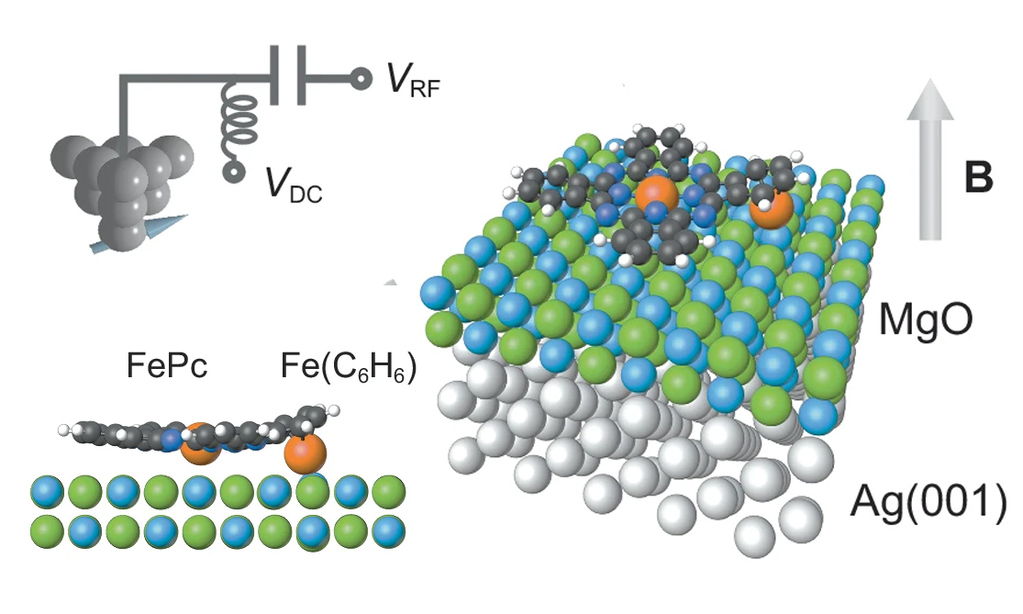
The design and control of atomic-scale spin structures constitute major challenges for spin-based quantum technology. In the journal Nature communications, Philip Willke and colleagues showcase a strategy for designing the quantum properties of molecular spin qubits by combining tip-assisted on-surface assembly with electron spin resonance scanning tunneling microscopy. They improve spin lifetime and coherent control by fabricating quantum ferrimagnets which are partly protected against inelastic electron scattering. Moreover, quantum ferrimagnets provide a versatile platform to study complex magnetic interactions.
read more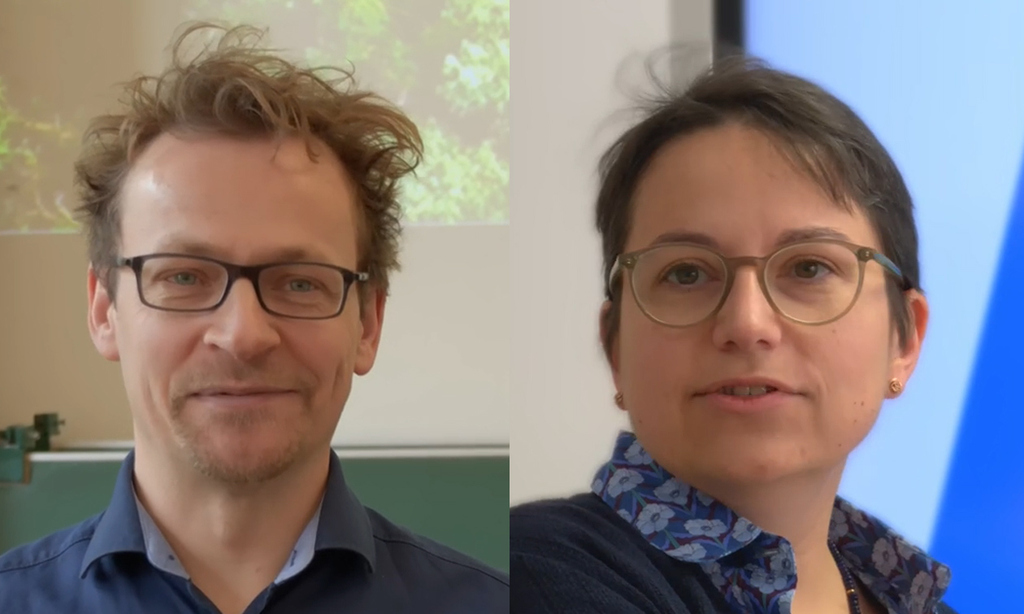
In 2025, two YIN members were honored with a KIT Faculty Teaching Award. Ulrike van der Schaaf received the award together with her colleagues Alexander Grünberger, Dirk Holtmann, and Jürgen Hubbuch from the KIT Department of Chemical and Process Engineering. They designed the lecture “Introduction to Bioengineering” linking theoretical content with practical application examples. Wilfried Liebig imparts solid engineering fundamentals and encourages students to develop creative solutions, which they can test in competitions. For his pioneering teaching and motivating approach, the KIT Department of Mechanical Engineering awarded him the Faculty Teaching Award.
Faculty Teaching Awards 2025mechanical%20Properties%20for%20All-Solid-State%20Batteries2_rdax_98p.jpg)
Solid-state batteries are based on inorganic solid electrolytes. They offer enhanced energy and power densities along with increased safety compared to state-of-the-art rechargeable batteries using liquid organic electrolytes. However, their stiffness and brittle nature can complicate cell fabrication and lead to the (chemo)mechanical failure during operation. In a study published in Chemistry of Materials, Florian Strauss and colleagues systematically investigated the effect of lithium iodide additives and the annealing temperature on phase composition and charge-transport properties. They show that glass-ceramic solid electrolytes achieve optimized ionic conductivity and the (chemo)mechanical properties, thus enabling long-term solid-state battery operation.
Chemistry of Materials